Will We Run Out of ML Data? Evidence From Projecting Dataset Size Trends
Based on our previous analysis of trends in dataset size, we project the growth of dataset size in the language and vision domains. We explore the limits of this trend by estimating the total stock of available unlabeled data over the next decades.
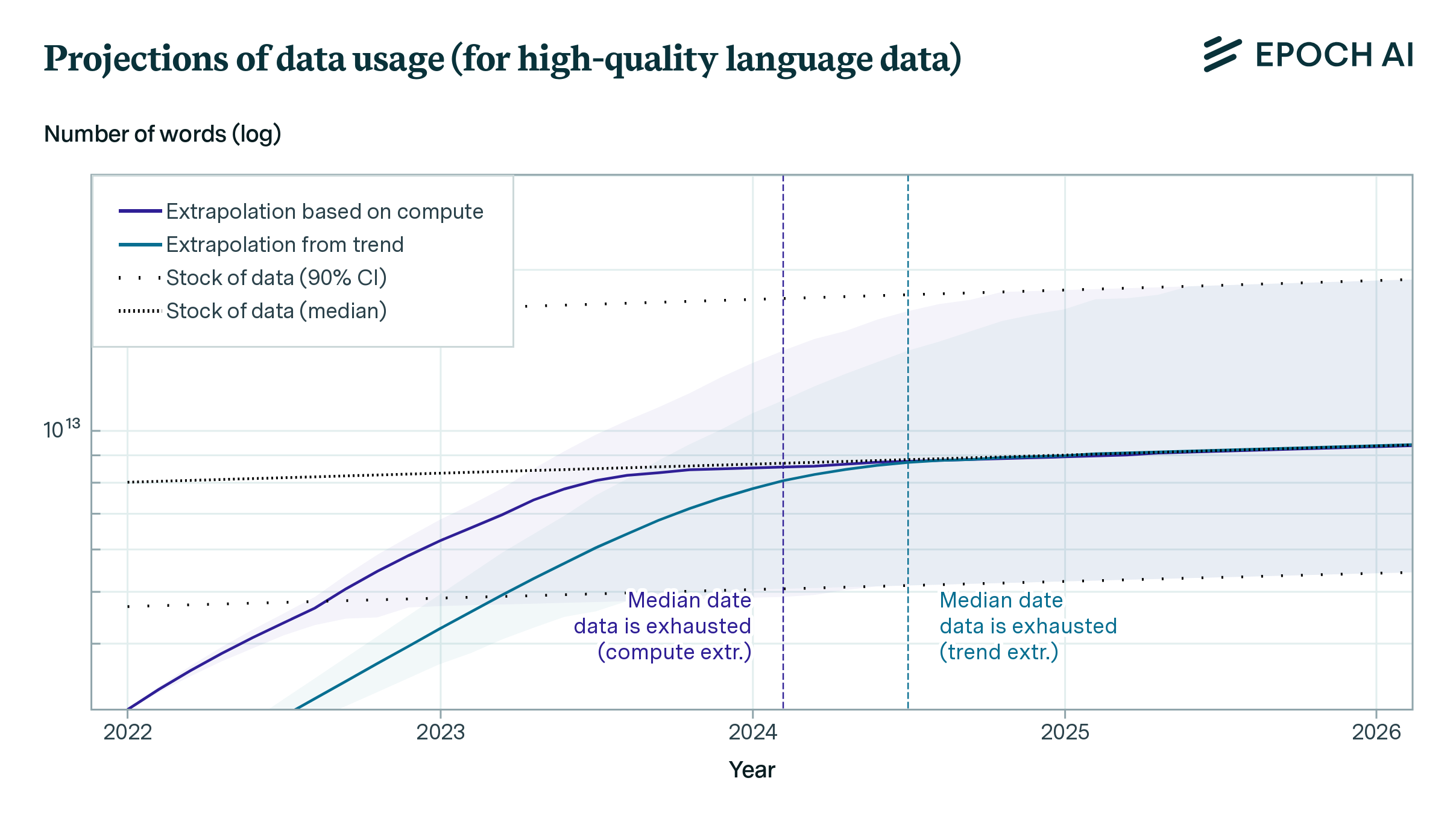
Published
Resources
This paper was originally published on Nov 10, 2022. For the latest research and updates on this subject, please see: Will We Run Out of Data? Limits of LLM Scaling Based on Human-Generated Data.
Our projections predict that we will have exhausted the stock of low-quality language data by 2030 to 2050, high-quality language data before 2026, and vision data by 2030 to 2060. This might slow down ML progress.
All of our conclusions rely on the unrealistic assumptions that current trends in ML data usage and production will continue and that there will be no major innovations in data efficiency. Relaxing these and other assumptions would be promising future work.
Figure 1: ML data consumption and data production trends for low quality text, high quality text and images.
Historical projection | Compute projection | |
Low-quality language stock | 2032.4
[2028.4 ; 2039.2] |
2040.5
[2034.6 ; 2048.9] |
High-quality language stock | 2024.5
[2023.5 ; 2025.7] |
2024.1
[2023.2 ; 2025.3] |
Image stock | 2046
[2037 ; 2062.8] |
2038.8
[2032 ; 2049.8] |
Table 1: Median and 90% CI exhaustion dates for each pair of projections.
Background
Chinchilla’s wild implications argued that training data would soon become a bottleneck for scaling large language models. At Epoch AI we have been collecting data about trends in ML inputs, including training data. Using this dataset, we estimated the historical rate of growth in training dataset size for language and image models.
Projecting the historical trend into the future is likely to be misleading, because this trend is supported by an abnormally large increase in compute in the past decade. To account for this, we also employ our compute availability projections to estimate the dataset size that will be compute-optimal in future years using the Chinchilla scaling laws.
We estimate the total stock of English language and image data in future years using a series of probabilistic models. For language, in addition to the total stock of data, we estimate the stock of high-quality language data, which is the kind of data commonly used to train large language models.
We are less confident in our models of the stock of vision data because we spent less time on them. We think it is best to think of them as lower bounds rather than accurate estimates.
Results
Finally, we compare the projections of training dataset size and total data stocks. The results can be seen in the figure above. Datasets grow much faster than data stocks, so if current trends continue, exhausting the stock of data is unavoidable. The table above shows the median exhaustion years for each intersection between projections.
In theory, these dates might signify a transition from a regime where compute is the main bottleneck to growth of ML models to a regime where data is the taut constraint.
In practice, this analysis has serious limitations, so the model uncertainty is very high. A more realistic model should take into account increases in data efficiency, the use of synthetic data, and other algorithmic and economic factors.
In particular, we have seen some promising early advances on data efficiency,1 so if lack of data becomes a larger problem in the future we might expect larger advances to follow. This is particularly true because unlabeled data has never been a constraint in the past, so there is probably a lot of low-hanging fruit in unlabeled data efficiency. In the particular case of high-quality data, there are even more possibilities, such as quantity-quality tradeoffs and learned metrics to extract high-quality data from low-quality sources.
All in all, we believe that there is about a 20%2 chance that the scaling (as measured in training compute) of ML models will significantly slow down by 2040 due to a lack of training data.
-
Eg, transformers with retrieval mechanisms are more sample efficient. Or see EfficientZero for a dramatic example, albeit in a different domain.
In addition to increased data efficiency, we have seen examples of synthetic data being used to train language models. ↩
-
This probability was obtained by polling some Epoch AI team members and taking the geometric mean of the results. ↩